Introduction
The term ‘artificial intelligence,’ is bandied about a great deal. Let’s take a few moments to find out what it is and how our world is changing because of it.
Artificial Intelligence (AI) refers to computer software that engages in humanlike activities, such as learning, planning and problem-solving. Artificial intelligence underpins most of what is going to happen in our new world. It’s been around for a while, and its importance will accelerate post-COVID-19. Intelligent machines are influencing nearly every facet of our lives to help improve efficiencies and augment our human capabilities.
A working understanding of AI is critical for just about every career because machines can learn and make decisions much quicker and more accurately than humans. There are many types of artificial intelligence, where instead of being programmed what to think, machines can observe, analyse and learn from data and mistakes just like our human brains do.
Financial institutions, law firms, media companies and insurance companies are all working on ways to use artificial intelligence to their advantage. AI is used to discover fraud, write news stories, and review legal contracts. No matter what industry you are now working in, AI is rapidly changing the way things get done.
Algorithms
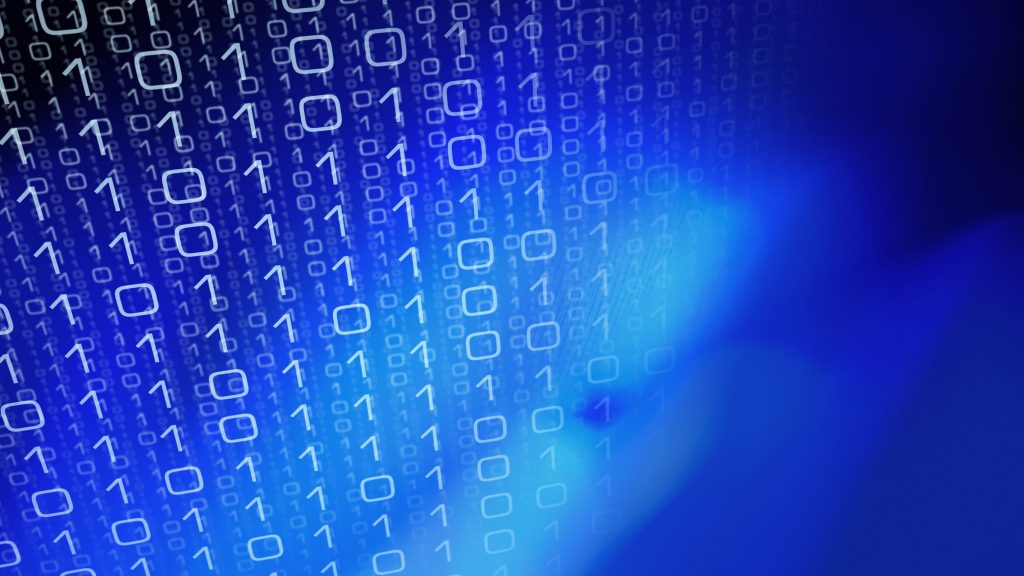
Algorithms are central to AI. An algorithm is a process or set of rules to be followed by a computer when doing calculations or solving problems. It’s pretty much the equivalent of a recipe to bake a cake. Except that the computer follows the exact set of rules every single time, without mistake. It is the set of instructions a computer will follow in order to complete a task.
Algorithms can be used to enable a machine to learn – in other words, solve problems, and not just execute instructions. This is known as machine learning. There are two types of machine learning: supervised learning and unsupervised learning.
Machine learning
An example of supervised learning is where a computer is given a large number of photographs. Along with the photographs is information about what is on them. The computer analyses, sorts, and interprets what is on the photographs. This is called training. You then give the computer new, unanalysed photographs without the accompanying information, and let it analyse, sort and interpret them, using the learning from the previous set.
In unsupervised learning, you give a computer a number of photographs of each of 5 different people. The computer must interpret the visual imagery to separate them into 5 different piles, one pile for each person. Unsupervised learning is useful for clustering, and there are many applications for customer segmentation in marketing.
Supervised learning can be used in sorting oranges to identify fruit that does not conform to a particular visual standard. It is also useful for decision support: a computer can be trained with large amounts of information about actual and historic stock market transactions. It can then be used to assess how effective a contemplated trade will be.
Predictive modelling algorithms use data and statistics to predict outcomes with data models. You can use this tool to predict the results in your favourite soccer league. Predictive modelling has many applications in online advertising and marketing. Modellers use web surfers’ historical data. They use algorithms to determine what kinds of products users might be interested in and what they are likely to click on.
Visualisation
Images and pictures are particularly difficult for a computer. You and I will look at a picture and see a tree, a cow and a house. The computer just sees a flat array of pixels. By itself, the computer has no sense of depth or perspective. In order for the software (convolutional neural networks to the savvy ones among you!) to interpret the flat pixel image, the computer compares the image with a very large set of image libraries. It goes back and forth between the libraries and the image. Hence the term convolutional. The algorithms initially look for and compare looking simple features such as edges and curves, and then build up to ‘see’ more abstract and complex concepts. When completed, the computer ‘sees’ the house or the cow as you and I would identify it. This is called visualisation. It brings a visual dimension (2D or 3D) to an object that is described in non-visual data. The visualisation allows an object to been “seen” without physically being there, with the help of these algorithms and convolutional neural networks.
You will immediately see how complex this is, and at the same time, you will see some of the practical applications. You might even have used facial recognition (one application of visualisation) to open your device to read this.
Deep learning
Deep learning is a type of machine learning where artificial neural networks learn from large amounts of data. They do this by themselves once the algorithms and neural networks are set up. In other words, the ‘machine’ can go ahead (provided it has enough data or images) and solve complex problems by itself. That is super cool!
Data
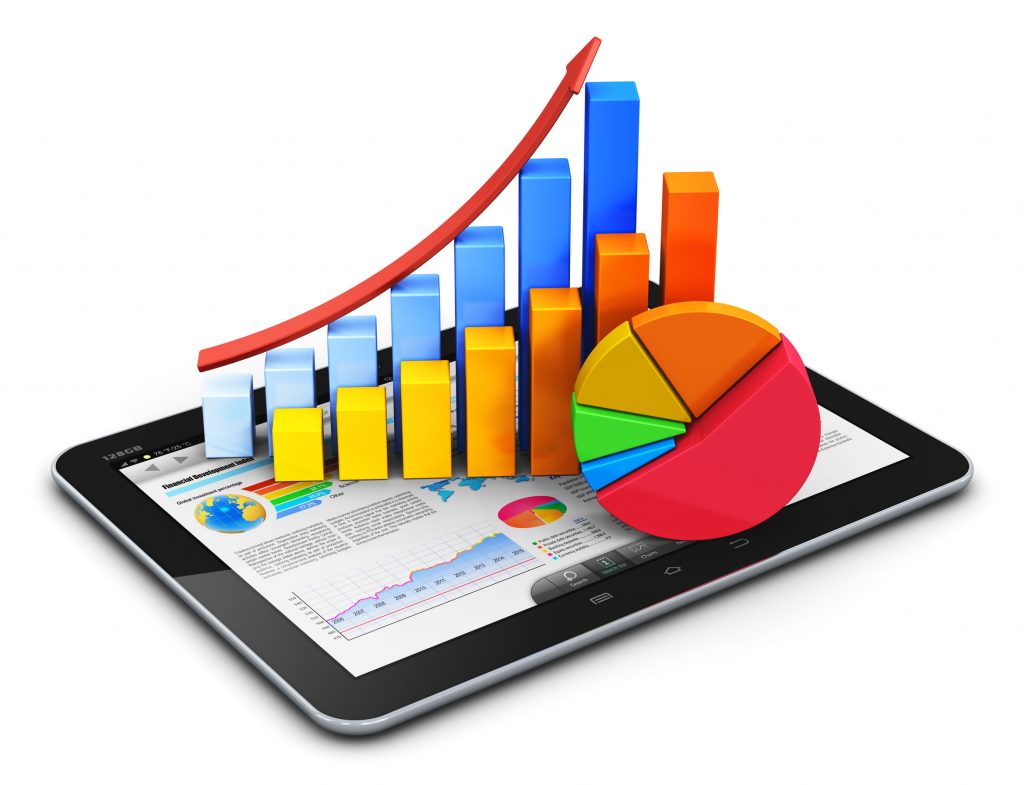
No conversation about Artificial Intelligence (AI) is complete without exploring the world of data and understanding how the data is processed. The characters or symbols manipulated by a computer are known as data. Data can be stored and transmitted in the form of electrical signals and recorded on magnetic or optical recording media. Big data is a huge collection of data and growing exponentially. Big data is so large and complex that none of the traditional data management tools is able to store it or process it efficiently.
Here are examples of big data:
More than 500 terabytes of new data go onto the databases of Facebook every day. A single jet engine can generate over 10 terabytes of data in 30 minutes of flight time. These amounts of data are mind-boggling. Big data challenges include capturing data, data storage, data analysis, search, sharing, transfer, visualisation, querying, updating, and information privacy.
Data that can be stored, accessed and processed in fixed format form are called ‘structured’ data. A typical example is employee records in a database. The details of names, salaries and contact details are structured in the database. It is easier to extract meaning from structured data.
Any data with an unknown form or structure is called ‘unstructured’ data. The millions of results in a Google search provides unstructured data. It is difficult to draw out meaning from unstructured data.
Organisations have massive amounts of data available to them, but they have to derive value from it since this data is in its raw form or “unstructured”.
Analysing big data can spot business trends, prevent diseases, make sense of climate change and combat crime.
Zubair Maqsood put together this diagram to explain the relationship between Artificial Intelligence, Machine Learning and Deep Learning:
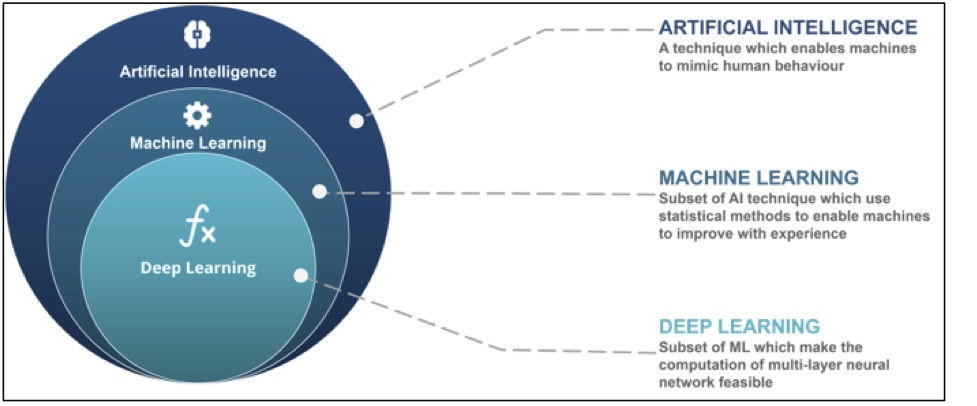
Now, after this very brief overview of the technology of artificial intelligence, let’s explore how it is changing our world.
Artificial intelligence and business today
The big advantage of AI is that it can process and analyse vast amounts of information, very quickly, much quicker than a human being can. Through the learning processes discussed above, it can compile options for decision making by humans.
An artificial intelligence application makes complex decisions on its own without human intervention. Its self-learning capability enables it to even take decisions in situations not foreseen by the programmers that created it. These capabilities allow artificial intelligence to be applied in many industries; whether it’s simply a chatbot answering first level customer queries or monitoring the blades and generators of a forest of wind turbines.
Machine learning is best in systems that capture vast amounts of data. For example, smart energy management systems collect data from sensors fixed to various assets like generators, transmission lines or factory plant. Machine learning algorithms then contextualise, analyse and process this huge flood of data. Human beings then review this output and take decisions for optimal energy usage and maintenance demands.
AI finds powerful applications in marketing. AI-powered tools trawl over a company’s website for blog articles, case studies, white papers, e-books, videos, etc. AI is then able to predict what product information will appeal to each identified market segment. Potential customers are then engaged via email, web, social, and mobile channels. The company can then undertake focused one-to-one value marketing on a scale unknown before AI.
Banks use AI for customer identification and authentication. Voice assistants and chatbots free front-line staff from routine queries and requests. AI analyses clients and provides personalised insights and product recommendations which deepen customer relationships. AI is also used to detect and prevent payments fraud and to improve processes for anti-money laundering and regulatory FICA checks.
In manufacturing, ongoing maintenance of production line machinery and equipment represents a major expense. Predictive maintenance is a vital technique in keeping these costs as low as possible. Predictive maintenance uses advanced AI algorithms in the form of machine learning and artificial neural networks to predict what plant and equipment may break down. This allows for a drastic reduction of costly unplanned downtime, as well as for extending the remaining useful life of plant and equipment.
The future of Artificial Intelligence
There is still much debate about the benefits and disadvantages of AI. A strong theme is that artificial intelligence will amplify human effectiveness but also threaten human autonomy, agency and capabilities. Computers may equal or even surpass human intelligence and capabilities on tasks such as complex decision-making, reasoning and learning, sophisticated analytics and pattern recognition, visual acuity, speech recognition and language translation. “Smart” systems in communities, in vehicles, in buildings and utilities, on farms and in business processes will save time, money and lives and offer opportunities for individuals to enjoy a more-customised future.
There is a great deal of optimism about AI in health care. AI will be extremely helpful in diagnosing and treating patients or helping senior citizens live fuller and healthier lives. AI’s ability to make sense of massive amounts of data will improve broad public-health programmes, from personal genomes to nutrition. AI will also make significant changes in formal and informal education systems.
But concerns still remain. People are worried about the loss of humanity in the workplace, the loss of jobs and the daunting challenge of learning new skills. There are concerns about the loss of privacy.
The Pew Research Centre investigated this and their findings on the threats and remedies are outlined in the table below.
AI and the future of humans: Experts express concerns and suggest solutions
CONCERNS | Human agency: Individuals are experiencing a loss of control over their lives |
Decision-making on key aspects of digital life is automatically ceded to code-driven, “black box” tools. People lack input and do not learn the context about how the tools work. They sacrifice independence, privacy and power over choice; they have no control over these processes. This effect will deepen as automated systems become more prevalent and complex. |
Data abuse: Data use and surveillance in complex systems is designed for profit or for exercising power |
Most AI tools are and will be in the hands of companies striving for profits or governments striving for power. Values and ethics are often not baked into the digital systems making people’s decisions for them. These systems are globally networked and not easy to regulate or rein in. | |
Job loss: The AI takeover of jobs will widen economic divides, leading to social upheaval |
The efficiencies and other economic advantages of code-based machine intelligence will continue to disrupt all aspects of human work. While some expect new jobs will emerge, others worry about massive job losses, widening economic divides and social upheavals, including populist uprisings. | |
Dependence lock-in: Reduction of individuals’ cognitive, social and survival skills |
Many see AI as augmenting human capacities but some predict the opposite – that people’s deepening dependence on machine-driven networks will erode their abilities to think for themselves, take action independent of automated systems and interact effectively with others. | |
Mayhem: Autonomous weapons, cybercrime and weaponized information |
Some predict further erosion of traditional socio-political structures and the possibility of great loss of lives due to accelerated growth of autonomous military applications and the use of weaponized information, lies and propaganda to dangerously destabilize human groups. Some also fear cybercriminals’ reach into economic systems. | |
SUGGESTED SOLUTIONS | Global good is No. 1: Improve human collaboration across borders and stakeholder groups |
Digital cooperation to serve humanity’s best interests is the top priority. Ways must be found for people around the world to come to common understandings and agreements – to join forces to facilitate the innovation of widely accepted approaches aimed at tackling wicked problems and maintaining control over complex human-digital networks. |
Values-based system: Develop policies to assure AI will be directed at ‘humanness’ and common good |
Adopt a ‘moon-shot mentality’ to build inclusive, decentralized intelligent digital networks ‘imbued with empathy’ that help humans aggressively ensure that technology meets social and ethical responsibilities. Some new level of regulatory and certification process will be necessary. | |
Prioritize people: Alter economic and political systems to better help humans ‘race with the robots’ |
Reorganize economic and political systems toward the goal of expanding humans’ capacities and capabilities in order to heighten human/AI collaboration and staunch trends that would compromise human relevance in the face of programmed intelligence. |
In conclusion
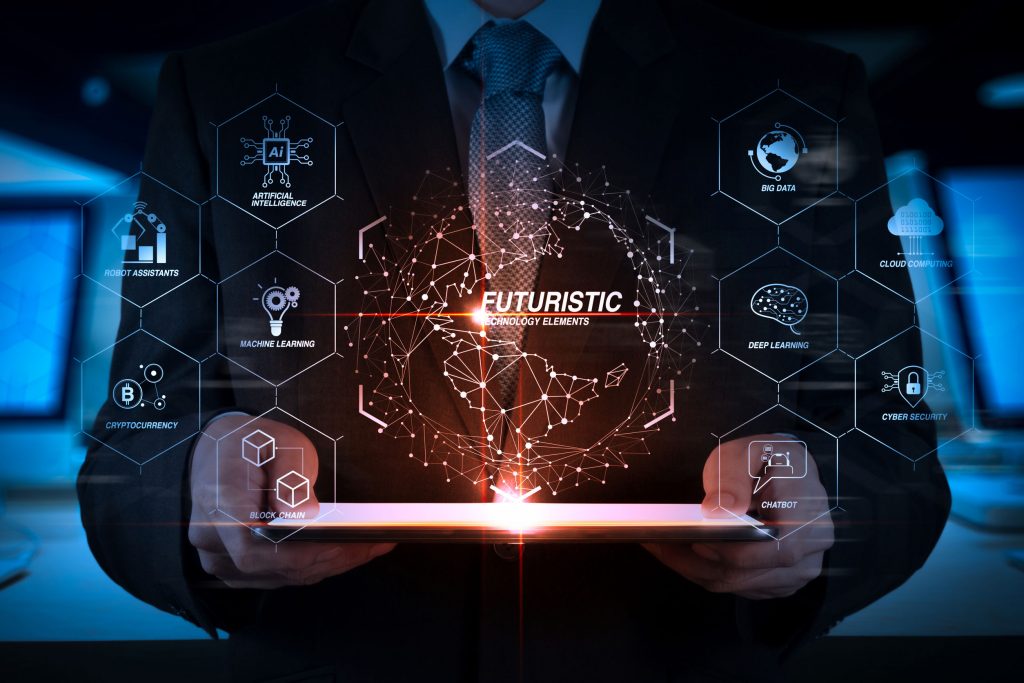
The onward march of artificial intelligence is inescapable. The future AI world will give all of us access to sophisticated information – about ourselves, our work and our society.
We are going to need governance structures to ensure that this information is used smartly, wisely and for our benefit. This will present challenges to those who have custody of this knowledge.
The best of AI is still to come. But it’s coming, and we are already adapting to it. The future is AI-enabled and ours is the privilege to live in these times.
Read also: The Fifth Industrial Revolution (5IR) and how it will change the business landscape