In today’s fast-changing digital world, businesses everywhere need to use and understand data effectively. As information floods daily, companies rely on data science and analytics to make informed decisions and stay ahead of the competition. If you’re considering a career in data or aiming to boost your business operations, it’s crucial to understand how these two fields differ and overlap.
This article will provide a breakdown of data analytics vs data science, outlining the meaning of data science and data analytics, their fundamental differences, and the education and skills required for each.
Table of Contents
- What Is Data Science?
- What Is Data Analytics?
- Data Science Vs. Data Analytics: Education And Work Experience
- What Does A Data Scientist Do?
- What Does A Data Analyst Do?
- Difference Between Data Scientist And Data Analyst: Roles And Responsibilities
- Data Scientist Vs. Data Analyst: Skill Comparison
- Salary Difference: Data Science Vs. Data Analytics
- Similarities Between Data Scientists And Data Analysts
- Conclusion
- Data Science vs Data Analytics – FAQs
What Is Data Science?
Data science is a field that focuses on finding valuable information from large amounts of data. It combines knowledge from statistics, computer science, and specific industries to analyse organised and unorganised data. As more companies rely on data to make decisions, data science has become crucial for staying competitive. It helps businesses predict future trends, improve operations, and customise products or services to meet customer needs better. As the demand for data insights grows, data scientists’ roles are becoming more critical in today’s data-driven world.
Data scientists use data mining, machine learning, and predictive analysis to discover patterns and trends that help make critical business decisions. This process covers everything from gathering and cleaning data to analysing and presenting it, making data science a powerful tool for solving complex problems. Because of this, data science plays a key role in shaping business strategies and driving innovation.
What Is Data Analytics?
Data analytics is examining and understanding data to gain insights that help businesses make informed decisions. Data analytics mainly analyses past data to identify trends, patterns, and connections. This helps organisations improve their operations and make better decisions based on a clear understanding of past performance.
Data analytics is crucial for businesses to boost their performance and increase profits. Many techniques and processes in this field have been automated, enabling quick data analysis through software and tools. By applying these methods, organisations can uncover insights that drive growth and innovation, ultimately enhancing their overall performance and competitiveness.
Data Science Vs. Data Analytics: Education And Work Experience
If you’re thinking about a career in data science or data analytics, it’s important to understand how these two fields differ. Both rely on strong math and statistics skills but require different types of education and work experience. Let’s take a look at the comparison between Data Science Vs. Data Analytics in terms of education and experience:
Education
To become a data scientist, you usually need a master’s or PhD in computer science, statistics, or engineering. Educational programmes like Postgraduate Diploma in Data Science often start by defining data science’s meaning, then proceed to provide a solid foundation for further study. The coursework is advanced to cover topics like machine learning, programming, and big data tools like Hadoop and Spark. Data scientists must also be skilled in languages like Python and R and understand complex algorithms.
Get detailed information about how to become a data scientist in South Africa.
Data analytics is often more accessible. A bachelor’s degree in Business, Economics, or Mathematics is usually sufficient. The focus is on learning Statistics, Data Management, and Basic Programming. Tools like Excel and SQL are commonly used, and some knowledge of Python or R is also helpful. This education helps data analysts turn data into actionable business insights.
Work Experience
Data scientists typically start their careers with significant prior experience or advanced education. They often work on complex projects involving programming and machine learning from the beginning. Internships or academic projects can help build the skills needed. Keeping up with the latest technology and methods in AI and machine learning is crucial.
Data analysts often start their careers right after finishing their undergraduate studies. They focus on tasks like data cleaning and basic statistical analysis. As they gain experience, they may handle more complex tasks and learn tools like Tableau or PowerBI. Their job involves interpreting data to support business decisions.
What Does A Data Scientist Do?
In today’s data-driven environment, data scientists are essential in turning raw data into useful insights that guide decision-making across various industries. Their role combines technical skills with business knowledge, allowing them to handle and analyse complex data effectively. Here’s an overview of the key responsibilities of a data scientist:
- Data Collection: Gathering data from different sources to create a complete dataset for analysis.
- Data Cleaning: Preparing and refining data by removing errors and inconsistencies to ensure it is accurate and ready for analysis.
- Analysis and Modeling: Using statistical techniques and machine learning algorithms to discover patterns and trends in the data.
- Collaboration: Working with various teams to incorporate data insights into strategic planning and decision-making processes.
- Visualisation: Designing clear and informative charts, dashboards, and reports to present findings and support business decisions.
What Does A Data Analyst Do?
Data analysts are crucial in transforming raw numbers and statistics into realistic interpretations that drive business decisions. They follow a structured approach to collecting, cleaning, analysing, and sharing data to support informed choices. Here’s a brief overview of their main responsibilities:
- Gather, Collect, and Interpret Data: Analysts start by collecting relevant information from various sources, such as websites, sensors, and social media. They must then understand and ensure the quality of this data, often collaborating with IT, sales, and marketing teams.
- Clean, Organise, and Validate Data: Analysts prepare data for analysis by fixing errors, managing missing values, removing outliers, and organising it properly. Proficiency in Excel, SQL, Python, and R tools is essential.
- Extract Actionable Insights: Analysts use statistical methods, machine learning, and visualisation tools to identify patterns and trends. Techniques such as regression analysis and predictive modelling help answer key business questions.
- Communicate Findings: After analysis, analysts present their results through interactive dashboards, detailed reports, and visualisations using tools like Tableau and Power BI. Clear communication ensures that insights are easily understood and actionable.
Difference Between Data Scientist And Data Analyst: Roles And Responsibilities
Understanding the differences between data scientists and data analysts is crucial for anyone looking to build a career in data. Data analysts examine existing data to spot trends and help make business decisions, while data scientists develop advanced algorithms and models to improve data processing and automation. Here’s a clearer comparison of their roles:
Aspect | Data Analyst | Data Scientist |
Responsibilities | Data analysts organise and clean data, identify patterns and share their findings using tools such as SQL, R, Power BI, and Tableau. | Data scientists develop algorithms, build systems, and create data structures to improve how data is collected and analysed. |
Skills and Tools | Data analysts use statistical tools and visualisation software to interpret data and clearly communicate the results. | Data scientists use programming languages and advanced techniques to build models that predict outcomes and enhance data analysis. |
Role Overlap | Data analysts use tools created by data scientists to discover insights and support business decision-making. | While data scientists often begin with data analysis, their primary focus is creating tools and systems for processing data. |
Soft Skills | Data analysts need good communication skills to explain their findings simply and understandably. | Data scientists require strong communication, business understanding, and problem-solving skills to address challenges and predict future trends. |
Data Scientist Vs. Data Analyst: Skill Comparison
When we look at the comparison between data scientists and data analysts, it’s essential to understand how their expertise and skills differ. Both roles involve working with data to derive insights, but their skills and approaches vary. Data scientists generally use advanced technologies and complex methods, while analysts use fundamental tools to support business decisions. Here’s a detailed comparison of the skills required for each role:
Skills for Data Scientists
A data scientist solves challenging problems and finds valuable interpretations in large datasets, helping to guide decisions. They need technical skills, strong analytical thinking, and a good understanding of their field to do this well. These skills help them make sense of data, uncover hidden patterns, and identify trends that drive new ideas and progress. Some of these skills can be listed as:
- Programming: Data scientists need to be proficient in programming languages like Python, R, and sometimes Java or Scala. This expertise is crucial for managing large datasets and performing complex analyses.
- Machine Learning and Predictive Modeling: Data scientists should be skilled in machine learning techniques to build predictive models that analyse large datasets and forecast future outcomes.
- Big Data Technologies: Data scientists must have experience with big data platforms such as Apache, Hadoop, and Spark, which are often required to handle and analyse large datasets.
- Innovation and Problem-Solving: Data scientists must be innovative and capable of developing new methods for data analysis and problem-solving, often creating new tools and techniques.
- Artificial Intelligence: Data scientists must possess knowledge of AI, including deep learning and neural networks, which are becoming increasingly crucial for crafting advanced data solutions.
- Advanced Statistical Analysis and Mathematics: Data scientists require a deep understanding of statistics and mathematics to create and apply complex algorithms and models for predicting, classifying, and forecasting data.
- Communication and Storytelling: Data scientists must communicate effectively, as they need to present their findings in a way that influences strategic decisions and drives innovation.
Skills for Data Analysts
A data analyst focuses on interpreting data to provide actionable information. To succeed in this role, they need strong analytical skills, attention to detail, and proficiency with data tools. These skills help them make sense of data, identify trends, and generate reports that drive informed decisions. Some of these skills include:
- Data Visualisation: Data analysts must use tools like Tableau, PowerBI, or advanced Excel features to create visual data representations that help make business decisions.
- Statistical Analysis and Mathematics: Data analysts should have a firm grasp of statistics and mathematics, which is necessary for accurately interpreting and analysing data.
- Data Manipulation and Analysis Tools: Data analysts must have knowledge of SQL for database queries, Excel for spreadsheets, and essential statistical software like R or Python, which are necessary for data analysis.
- Reporting: Data analysts must be skilled in preparing clear, detailed reports that effectively communicate their findings to stakeholders.
- Business Acumen: Data analysts must understand the business context, and knowing which data is relevant to solving business problems is crucial.
- Communication: Data analysts should possess strong verbal and written communication skills, which are essential for explaining insights and findings to team members and stakeholders who may need a technical background.
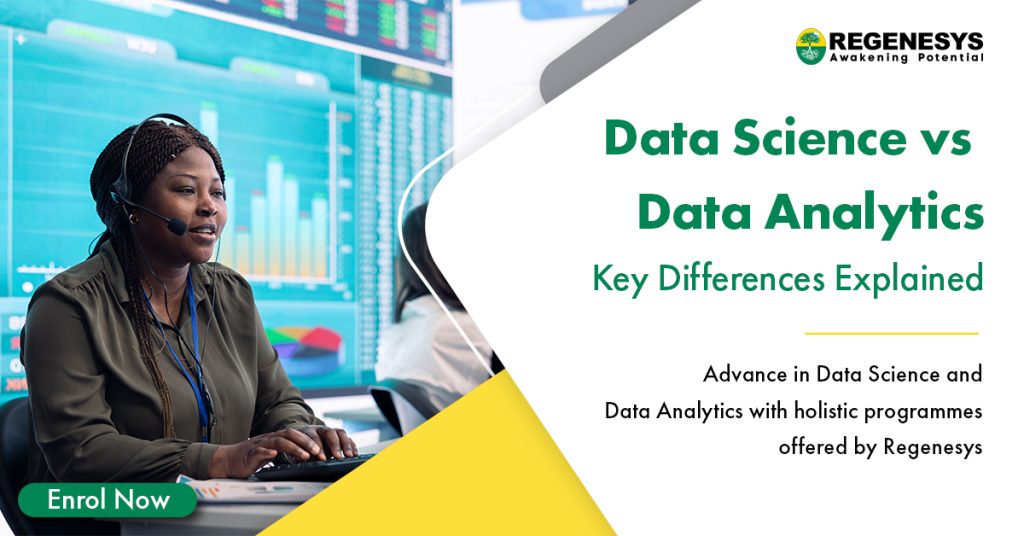
Salary Difference: Data Science Vs. Data Analytics
There’s a notable salary gap between data scientists and data analysts in South Africa. A Data Scientist typically has more advanced skills, handles more complex tasks, and earns about R850,795 a year. This high salary reflects their expertise in machine learning and data modelling.
In contrast, a Data Analyst who collects and analyses data to help with decision-making earns around R325,380 annually. This lower salary reflects the more basic skills and responsibilities of the role.
Similarities Between Data Scientists And Data Analysts
Although data scientists and analysts have different roles, they share several critical skills and responsibilities. Both are essential in helping businesses make smart, data-driven decisions by analysing and understanding data. Here are some key similarities between the two:
- Data-Driven Decision Making: Both data scientists and data analysts help businesses make better decisions by using data to provide valuable insights.
- Programming Knowledge: Both data scientists and analysts require knowledge of programming languages like SQL, R, Python, and Java, though the specific languages and tools they use might differ.
- Statistical Skills: Both data scientists and analysts need a good understanding of statistics. However, data scientists usually need more advanced statistical knowledge.
- Programming Skills: While both data scientists and data analysts are involved in programming, the languages and tools used can vary depending on the specific tasks and goals of each.
Conclusion
In conclusion, when evaluating data science vs data analytics, it’s crucial to understand the different roles each plays in managing data. Data scientists build complex models and algorithms to find deep insights and predict future trends, which requires advanced degrees and skills in machine learning and big data tools. Meanwhile, analysts analyse past data to spot patterns and trends using visualisation tools and statistical methods. Understanding the breakdown of data analytics vs data science helps clarify how these roles differ and overlap. Both are vital for businesses to use data effectively, drive innovation, and improve their strategies.
Ready to master data skills? Explore Regenesys School of Technology’s Bachelor of Science in Computer Science & Postgraduate Diploma in Data Science programmes to excel in both fields and advance your career. Visit our website today!
Data Science vs Data Analytics – FAQs
What’s the difference between data science and data analysis?
Data science focuses on creating complex models and algorithms to predict future trends, while data analytics looks at past data to find patterns and trends.
What does a data scientist do?
Data scientists collect, clean, and analyse data and use advanced techniques to create models that predict future outcomes.
What does a data analyst do?
Data analysts gather and clean data, then analyse it to provide insights that help businesses make better decisions.
What education is needed for data science vs data analytics?
Data scientists usually need a master’s or PhD in computer science or statistics, while data analysts generally need a bachelor’s degree in business, economics, or mathematics.
How do salaries compare for data scientists and data analysts?
Data scientists typically earn more than data analysts. Data scientists make about R850,795 annually, while analysts earn around R325,380 annually.
3 Comments
hiii team
hi
hi