Today, most companies depend on data-driven decision-making to stay relevant and competitive in their industry. This reliance has brought two key fields into the spotlight: Applied Statistics and Data Science. While both are important in transforming raw data into actionable insights, they approach this task from different angles.
For instance, Applied Statistics uses statistical methods to solve real-world problems, while Data Science integrates programming, machine learning, and domain-specific knowledge. Hence, if you are considering a career in either of these fields, it is important that you know their differences.
In this article, we will discuss Applied Statistics vs Data Science, exploring their differences, similarities and connections. We will also explore their job opportunities and salary differences, helping you to choose the right field that matches your career goals and interests.
Table of Contents
- What is Data Science?
- What is Applied Statistics?
- Differences between Applied Statistics and Data Science
- Similarities Between Applied Statistics and Data Science
- Top Real-World Applications of Applied Statistics and Data Science
- Top Job Roles For Applied Statistics and Data Science Aspirants
- Applied Statistics vs Data Science – Salary Difference
- Applied Statistics vs Data Science: Which One Should You Choose?
- Conclusion
- Applied Statistics vs Data Science – FAQs
What is Data Science?
Data Science is the process of extracting valuable insights from large and complex data sets. It involves several key disciplines, including Statistics, Artificial Intelligence, Mathematics, And Computer Science, to analyse vast data and find patterns. By identifying these patterns and trends, Data Science helps organisations make informed decisions, forecast trends, and identify opportunities for growth.
Moreover, data scientists use a range of tools and techniques, such as Python, R, and Tableau, to clean, integrate, visualise, and statistically analyse data. As you are trying to learn about Applied Statistics vs Data Science, you must know that data science can drive innovation and efficiency across various sectors through its deeper insights and broader applications.
What is Applied Statistics?
Applied Statistics involves the collection, analysis, and interpretation of data to identify patterns, eliminate biases, and aid in decision-making. This field is integral to business intelligence, helping organisations understand performance and find opportunities. It also includes evaluating commercial data to improve product lines and assess employee performance.
While statistical analysis is often used in data analytics, it goes further by carefully checking and confirming patterns and correlations to ensure their accuracy. Hence, when it comes to Applied Statistics vs Data Science, applied statistics focuses on rigorous data analysis and validation to ensure accurate interpretation and decision-making.
Differences between Applied Statistics and Data Science
When exploring Applied Statistics vs Data Science, you must know how each field differs. The table below highlights the key distinctions between these two disciplines regarding their scope, methodologies, problem-solving approaches, and data handling. So, let us take a look at the difference between applied statistics and data science:
Aspects | Applied Statistics | Data Science |
Scope and Focus | Focuses on analysing data to answer specific research questions or practical problems within a domain. | It encompasses statistics, computer science, machine learning, and domain expertise to extract insights from complex datasets. |
Methodologies and Tools | Utilises traditional statistical software like SAS, SPSS, and Stata for analysis and modelling. | Employs programming languages (Python, R, SQL) and tools (TensorFlow, scikit-learn, Apache Spark) for data analysis, machine learning, and big data processing. |
Problem-solving Approach | Hypothesis-driven, using statistical tests to validate theories and hypotheses. | Exploratory and iterative, using techniques like data visualisation, feature engineering, and model tuning to refine predictive models. |
Domain Expertise | Often requires deep domain knowledge to design studies and interpret results specific to a field. | Can work across multiple domains, applying analytical skills to a wide range of problems. |
Data Handling | Primarily deals with well-structured and organised datasets, focusing on mathematical models and statistical analysis. | Handles large, diverse datasets, including structured and unstructured data, focusing on data cleaning, integration, and feature engineering. |
Also, find out the difference between data science and data mining
Similarities Between Applied Statistics and Data Science
As you understand the Applied Statistics vs Data Science landscape, you must also know their shared aspects. Despite their different scope and focus, both these fields use fundamental techniques and principles to analyse and interpret data. Given below are some of the key similarities between applied statistics and data science:
- Predictive Modelling Approaches: Predictive modelling is a core component in applied statistics and data science that forecasts future trends and outcomes. While Data Science uses machine learning models, Applied Statistics employs regression models to achieve similar predictive goals.
- Data Analysis Techniques: Applied Statistics and Data Science use methods like exploratory data analysis, hypothesis testing, and regression modelling to identify patterns and insights from data.
- Experiment Design Strategies: In the Applied Statistics vs Data Science landscape, both fields involve designing experiments to test hypotheses and validate results, whether through randomised controlled trials in Applied Statistics or A/B testing in Data Science.
- Mathematical Foundations: Both fields are built on a strong mathematical framework, including concepts from probability theory, calculus, linear algebra, and statistical inference.
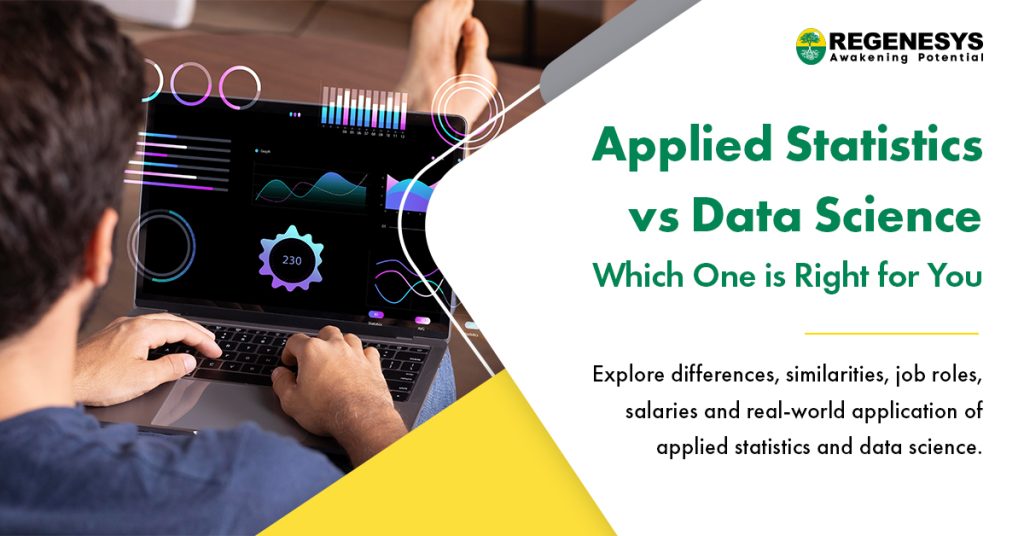
Top Real-World Applications of Applied Statistics and Data Science
Both applied statistics and data science offer valuable insights and solutions across various industries. Hence, when you discuss Applied Statistics vs Data Science, understanding how these fields are utilised in real-world scenarios is essential. Here, we will explore the key applications of each field:
Data Science Real-World Applications
Since data science uses advanced computational techniques and big data tools, it offers applications across various industries like the following:
- Healthcare: Analyses clinical data trends to forecast illnesses and optimise patient treatment.
- Retail and E-Commerce: Personalises customer experiences and improves sales through data analysis.
- Banking and Finance: Detects fraud and manages risk through predictive analytics and customer segmentation.
Applied Statistics Real-World Applications
By implementing rigorous mathematical models, applied statistics focus on interpreting data for the following areas:
- Stock Market Analysis: Determines dynamic stock values to assist in financial planning and investment decisions.
- Weather Forecasting: Uses probability and statistical models to predict weather conditions accurately.
- Sports Analytics: Compares player and team performances to inform game strategic decisions.
Top Job Roles For Applied Statistics and Data Science Aspirants
In the debate on the career aspect of Applied Statistics vs Data Science, you must know the job roles in each field. Since both these fields offer a wide variety of career roles with distinct purposes and responsibilities, you must go for the right one that aligns with your career interests and goals. So, here are the job roles you will observe in Applied Statistics and Data Science:
Data Science Job Roles
Data science is highly essential in driving data-driven decision-making, optimising processes, and creating predictive models across numerous industries. As a result, you will find the following job positions in the data science field:
- Data Analyst
- Data Architect
- Data Engineer
- Database Manager
- Machine Learning Engineer
- Business Analyst
Know how to become a data scientist in South Africa
Applied Statistics Job Roles
Applied statistics professionals play a crucial role in helping organisations make data-driven decisions and solve complex problems. They design experiments, analyse data, and provide insights that guide business strategies and research outcomes. Here are some of the job roles in applied statistics:
- Market Research Analyst
- Financial Analyst
- Economist
- Statistical Consultant
- Quantitative Analyst
- Statistician
Applied Statistics vs Data Science – Salary Difference
While you understand the Applied Statistics vs Data Science landscape, you must know that in South Africa, data scientists typically earn higher salaries compared to applied statisticians. The average annual salary for a data scientist is R699,701, with an additional bonus of R33,376. However, entry-level data scientists make about R496,102, while those with 8+ years of experience can earn R875,896.
On the other hand, applied statisticians have an average annual salary of R666,914 and a bonus of R21,541. When the entry-level statisticians earn approximately R472,170, the senior statisticians make around R834,852.
Applied Statistics vs Data Science: Which One Should You Choose?
Choosing between Applied Statistics vs Data Science landscape must depend on your skills and career interests. For instance, data science suits those passionate about coding and business, requiring strong skills in computer science and mathematics, along with effective communication skills to explain technical details. It is ideal for those who enjoy working with large data sets and teamwork.
On the other hand, applied statistics is best for those interested in mathematical modelling and data analysis across various sectors. It is also a great fit for those who are ambitious about social justice and want to make a difference through their work.
Conclusion
Both Applied Statistics and Data Science offer compelling career paths with their own unique focus and skill requirements. Data Science depends on advanced computational techniques and machine learning, ideal for those passionate about coding and handling large data sets. It is well-suited for those who enjoy innovative problem-solving and teamwork. On the other hand, Applied Statistics focuses on rigorous mathematical models and data analysis, making it perfect for those interested in detailed, methodical work and its real-world applications. We hope this article has provided valuable insights into the Applied Statistics vs Data Science landscape, helping you to choose the field that best aligns with your skills and career goals.
Build your career with Regenesys School of Technology’s Postgraduate Diploma in Data Science and learn the skills you need to succeed in the data-driven world. Enrol now!
Applied Statistics vs Data Science – FAQs
How do Applied Statistics and Data Science contribute to business intelligence?
Both fields are vital to business intelligence. Applied Statistics aids data-driven decisions through rigorous analysis, while Data Science uses advanced algorithms and machine learning to predict trends and uncover deeper insights.
Which field has higher earning potential: Applied Statistics or Data Science?
Data Science generally offers higher earning potential compared to Applied Statistics. In South Africa, the average salary for a Data Scientist is higher than that of an Applied Statistician, reflecting the demand for data science skills in the industry.
Can I transition from Applied Statistics to Data Science?
Yes, transitioning from Applied Statistics to Data Science is possible by developing skills in programming, machine learning, and big data tools. Many statisticians do this to meet the growing demand in data science.
Which field offers better career opportunities: Data Science vs Applied Statistics?
Both fields offer strong career opportunities, but Data Science tends to have a broader scope due to its integration of advanced computational techniques, programming, and machine learning. This often leads to higher-paying roles, especially in tech-driven industries.
What are the common career paths in applied statistics vs data science?
In the statistics vs data science comparison, career paths in statistics include roles like Statistician and Market Research Analyst, while data science careers feature positions like Data Scientist and Machine Learning Engineer.